It is important to evaluate the accuracy and transparency when evaluating an AI stock trading prediction. This will allow you to understand how it makes predictions and also ensure that it matches your trading goals. Here are 10 methods to evaluate the model's transparency and the model's interpretability.
1. Review Documentation and Explainations
What's the reason? A thorough documentation explains how the model works as well as its limitations and how the model generates predictions.
How to find detailed reports or documentation that describes the model's structure. This covers data sources and preprocessing, and feature selection. Simple explanations will enable you understand the logic behind every prediction.
2. Check for Explainable AI (XAI) Techniques
Why: XAI techniques make models more readable by highlighting the elements that are most important.
How: Check to see whether your model is interpretable using software such as SHAP, or LIME. These can identify the significance of features and make individual forecasts.
3. Examine the contribution and importance of Specific Features
The reason is knowing which variables the models relies on most allows you to determine if they are focusing on relevant drivers of the market.
How do you find an index based on the contribution or importance scores of features. These show the ways in which each feature (e.g. price, volume and sentiment) impacts the outputs. This can help validate the logic behind the predictor.
4. Be aware of the model's complexity in relation to. interpretability
Why? Overly complex models can be difficult to understand. This may reduce your ability and your confidence to act upon predictions.
What should you do: Determine if the model you are considering is compatible with your requirements. More simple models (e.g. linear regression or decision tree) are generally preferred over black-box complex models (e.g. Deep neural networks).
5. Transparency is a must in the model parameters and also in hyperparameters
Why is this? Transparent hyperparameters provide insights into model calibration which can affect risk or reward biases.
How: Document the hyperparameters. This helps you understand your model's sensitivity. Then, you can adjust it accordingly for different market conditions.
6. Request Access to Backtesting Results and Actual-World Performance
Why: Transparent backtesting allows you to examine how your model performs under different market conditions. This gives you an idea of the model's accuracy.
How to go about reviewing backtesting reports that show metrics (e.g. Sharpe ratio, max drawdown) across multiple time periods and market phases. Seek out transparency for both profitable and inefficient periods.
7. Analyze the model's response to changes in the market
The reason: A model that adjusts itself to market conditions can provide more accurate forecasts, however it is important to know the reason and how it is affected when it alters.
Find out if a model has the ability to adapt to changing information (e.g. market cycles, bull and bear), as well as if a decision was made to change method or strategy. Transparency can help you understand how the model adjusts to new information.
8. Search for Case Studies or Examples of Model Decisions
Why: Example prediction will show the way a model responds to certain situations. This helps clarify its process of decision-making.
Ask for examples from past market scenarios. For instance how the model's response to recent news or earnings reports. The model's logic can be uncovered through thorough case studies.
9. Transparency of Data Transformations and Preprocessing
What is the reason? Because transformations (such as scaling, or encoded) can affect the interpretability of data by altering how input data appears in the model.
How to: Find documentation on preprocessing data steps such as feature engineering, normalization or other similar processes. Understanding the way these transformations operate will help you understand why the model prioritizes specific signals.
10. Check for models. Bias Disclosure and Limitations
What's the reason? All models have limitations. Knowing these helps you use the model more effectively without over-relying on its forecasts.
What to do: Read all disclosures regarding model biases. Transparent limits allow you to avoid overconfident trades.
If you focus your attention on these tips, it is possible to determine the accuracy and transparency of an AI model of stock trading predictions. This will allow you to gain confidence in the use of this model and also learn how predictions are made. Read the most popular https://www.inciteai.com/news-ai for blog examples including ai stock investing, ai in the stock market, stock market ai, new ai stocks, artificial intelligence companies to invest in, ai stock companies, artificial intelligence trading software, artificial intelligence stock picks, stock investment, stock market analysis and more.
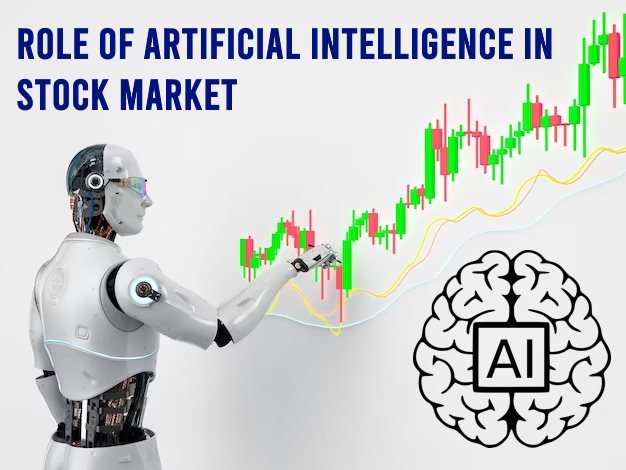
Ten Tips On How To Evaluate The Nasdaq By Using An Investment Prediction Tool
To analyze the Nasdaq Composite Index with an AI model for trading stocks it is important to know the unique characteristics of this index and components that are focused on technology as well as the AI model's ability to analyze and predict the index's movement. Here are 10 guidelines for evaluating the Nasdaq using an AI trading predictor.
1. Learn more about the Index Composition
Why? The Nasdaq composite comprises more than 3,000 companies, mostly in the technology, biotechnology and internet industries. This makes it different from an index with more diversification like the DJIA.
How to: Get acquainted with the largest and most influential corporations on the index. Examples include Apple, Microsoft, Amazon and many more. By recognizing their influence on the index, the AI model can be better able to predict the overall movement.
2. Incorporate specific industry factors
Why? The Nasdaq market is heavily affected by sector-specific and technology trends.
How to: Include relevant variables to your AI model, such as the performance of the tech sector, earnings reports or trends in the software and hardware sectors. Sector analysis increases the predictive power of the model.
3. Make use of Technical Analysis Tools
What are they? Technical indicators capture market mood and trends in price action on the most volatile Indexes like the Nasdaq.
How: Incorporate technical analysis tools like moving averages, Bollinger Bands, and MACD (Moving Average Convergence Divergence) into the AI model. These indicators will assist you to identify buy/sell signals.
4. Be aware of economic indicators that affect Tech Stocks
Why? Economic aspects, such as inflation, interest rates and employment, can affect the Nasdaq and tech stocks.
How: Incorporate macroeconomic indicators that apply to the tech sector, like consumer spending trends, tech investment trends and Federal Reserve policy. Understanding these relationships will help improve the model.
5. Evaluate the Impact of Earnings Reports
The reason is that earnings announcements from major Nasdaq-listed companies can cause price fluctuations and affect the index performance.
How: Make sure that the model follows earnings reports and adjusts forecasts to be in sync with the dates. It is also possible to improve the accuracy of prediction by analyzing the reaction of historical prices to earnings announcements.
6. Make use of the Sentiment analysis for tech stocks
The sentiment of investors has the potential to greatly affect prices of stocks. Especially in the field of technology, where trends can rapidly change.
How to: Include sentiment analysis of social media, financial reports, and analyst rating into the AI models. Sentiment metrics give context and can improve predictive abilities.
7. Perform backtesting of high-frequency data
Why: Because the volatility of the Nasdaq is well-known It is crucial to test your predictions with high-frequency trading.
How to: Utilize high-frequency data sets to backtest AI model predictions. This helps to validate its accuracy when compared to different market conditions.
8. The model's performance is evaluated through market volatility
The reason is that Nasdaq is susceptible to sharp corrections. Understanding how the model behaves during downturns, is essential.
Review the model's performance over time during significant market corrections or bear markets. Stress testing can reveal its durability and capability to limit losses during volatile periods.
9. Examine Real-Time Execution Metrics
How? Profits are dependent on efficient trade execution particularly when the index fluctuates.
How to track execution metrics, including fill rate and slippage. Examine how the model forecasts the best entry and exit points for Nasdaq related trades, ensuring that execution aligns with predictions.
Review Model Validation Using Out-of Sample Test
The reason: Testing the model on new data is crucial to make sure that it is able to be generalized effectively.
How do you conduct rigorous out of sample testing with historic Nasdaq Data that weren't used during training. Compare the predicted performance with actual performance to ensure accuracy and reliability.
These tips will aid you in assessing the validity and reliability of an AI predictive model for stock trading in analyzing and predicting the movements in Nasdaq Composite Index. Check out the most popular ai intelligence stocks examples for blog tips including top stock picker, best stock analysis sites, stock market prediction ai, ai for stock prediction, new ai stocks, artificial intelligence stock picks, best website for stock analysis, stock investment prediction, top ai companies to invest in, top ai companies to invest in and more.
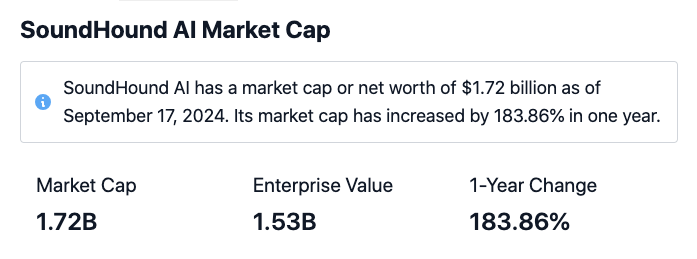